Section 6
Multiple Regression
By Boundless
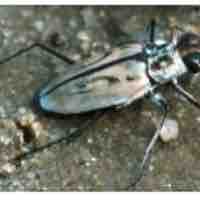
Multiple regression is used to find an equation that best predicts the
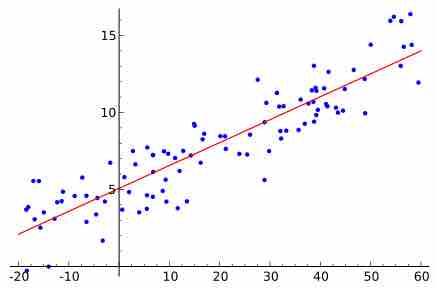
The purpose of a multiple regression is to find an equation that best predicts the
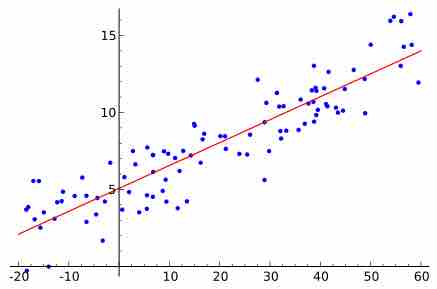
The results of multiple regression should be viewed with caution.
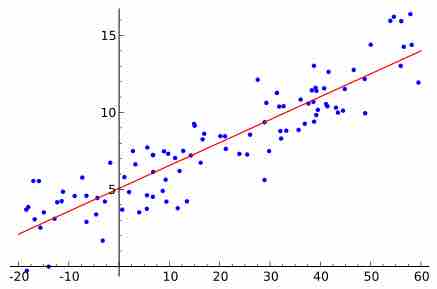
Standard multiple regression involves several independent variables predicting the dependent variable.
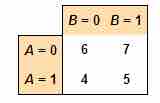
In regression analysis, an interaction may arise when considering the relationship among three or more variables.
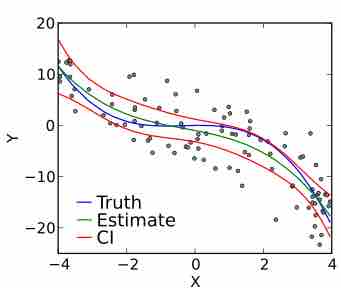
The goal of polynomial regression is to model a non-linear relationship between the independent and dependent variables.
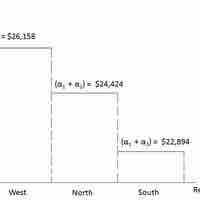
Dummy, or qualitative variables, often act as independent variables in regression and affect the results of the dependent variables.
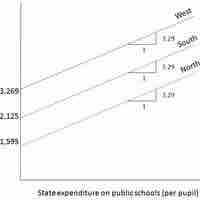
A regression model that contains a mixture of quantitative and qualitative variables is called an Analysis of Covariance (ANCOVA) model.
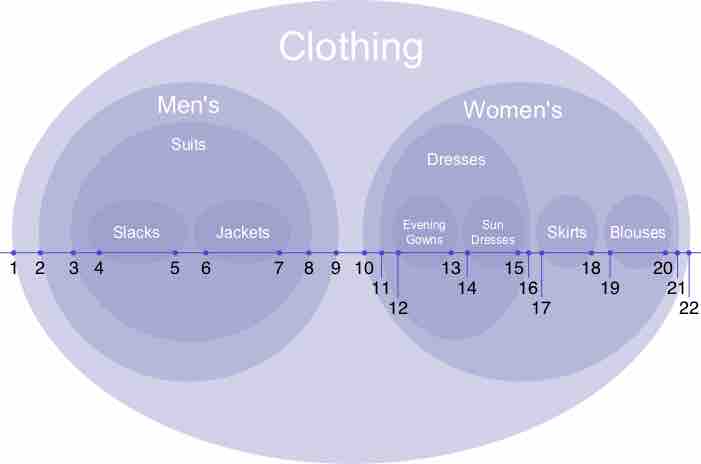
Multilevel (nested) models are appropriate for research designs where data for participants are organized at more than one level.
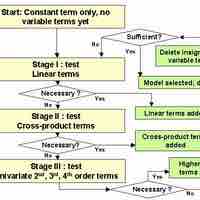
Stepwise regression is a method of regression modeling in which the choice of predictive variables is carried out by an automatic procedure.
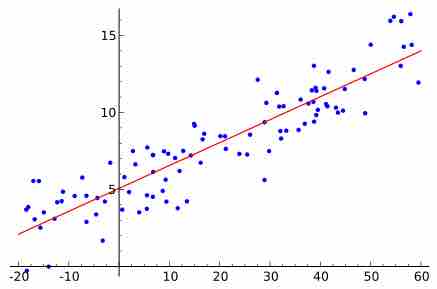
There are a number of assumptions that must be made when using multiple regression models.
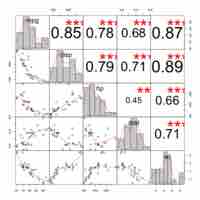
Some problems with multiple regression include multicollinearity, variable selection, and improper extrapolation assumptions.