Multiple regression is beneficial in some respects, since it can show the relationships between more than just two variables; however, it should not always be taken at face value.
It is easy to throw a big data set at a multiple regression and get an impressive-looking output. But many people are skeptical of the usefulness of multiple regression, especially for variable selection, and you should view the results with caution. You should examine the linear regression of the dependent variable on each independent variable, one at a time, examine the linear regressions between each pair of independent variables, and consider what you know about the subject matter. You should probably treat multiple regression as a way of suggesting patterns in your data, rather than rigorous hypothesis testing.
If independent variables
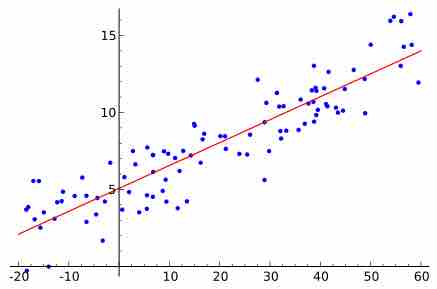
Linear Regression
Random data points and their linear regression.