When To Use Multiple Regression
You use multiple regression when you have three or more measurement variables. One of the measurement variables is the dependent (
Multiple Regression For Prediction
One use of multiple regression is prediction or estimation of an unknown
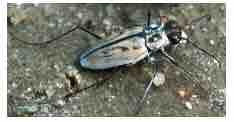
Atlantic Beach Tiger Beetle
This is the Atlantic beach tiger beetle (Cicindela dorsalis dorsalis), which is the subject of the multiple regression study in this atom.
Multiple Regression For Understanding Causes
A second use of multiple regression is to try to understand the functional relationships between the dependent and independent variables, to try to see what might be causing the variation in the dependent variable. For example, if you did a regression of tiger beetle density on sand particle size by itself, you would probably see a significant relationship. If you did a regression of tiger beetle density on wave exposure by itself, you would probably see a significant relationship. However, sand particle size and wave exposure are correlated; beaches with bigger waves tend to have bigger sand particles. Maybe sand particle size is really important, and the correlation between it and wave exposure is the only reason for a significant regression between wave exposure and beetle density. Multiple regression is a statistical way to try to control for this; it can answer questions like, "If sand particle size (and every other measured variable) were the same, would the regression of beetle density on wave exposure be significant? "
Null Hypothesis
The main null hypothesis of a multiple regression is that there is no relationship between the