A
While
Calculation From a Raw Score
A raw score is an original datum, or observation, that has not been transformed. This may include, for example, the original result obtained by a student on a test (i.e., the number of correctly answered items) as opposed to that score after transformation to a standard score or percentile rank. The
The conversion of a raw score,
where
A key point is that calculating
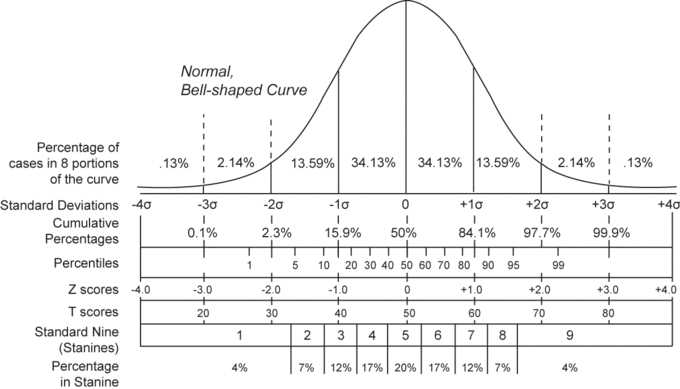
Normal Distribution and Scales
Shown here is a chart comparing the various grading methods in a normal distribution.