Required Sample Sizes for Hypothesis Tests
A common problem faced by statisticians is calculating the sample size required to yield a certain power for a test, given a predetermined Type I error rate
By Tables
The table shown in can be used in a two-sample
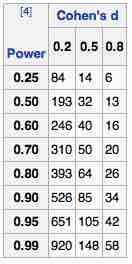
Sample Size Determination
This table can be used in a two-sample
The parameters used are:
- The desired statistical power of the trial, shown in column to the left.
- Cohen's
$D$ (effect size), which is the expected difference between the means of the target values between the experimental group and the control group divided by the expected standard deviation.
Mead's Resource Equation
Mead's resource equation is often used for estimating sample sizes of laboratory animals, as well as in many other laboratory experiments. It may not be as accurate as using other methods in estimating sample size, but gives a hint of what is the appropriate sample size where parameters such as expected standard deviations or expected differences in values between groups are unknown or very hard to estimate.
All the parameters in the equation are in fact the degrees of freedom of the number of their concepts, and hence, their numbers are subtracted by 1 before insertion into the equation. The equation is:
where:
-
$N$ is the total number of individuals or units in the study (minus 1) -
$B$ is the blocking component, representing environmental effects allowed for in the design (minus 1) -
$T$ is the treatment component, corresponding to the number of treatment groups (including control group) being used, or the number of questions being asked (minus 1) -
$E$ is the degrees of freedom of the error component, and should be somewhere between 10 and 20.
By Cumulative Distribution Function
Let
and an alternative hypothesis:
for some "smallest significant difference"
- reject
$H_0$ with a probability of at least$1-\beta$ when$H_a$ is true (i.e., a power of$1-\beta$ ), and - reject
$H_0$ with probability$\alpha$ when$H_0$ is true,
then we need the following:
If
and so "reject