Tests of significance are a statistical technology used for ascertaining the likelihood of empirical data, and, from there, for inferring a real effect, such as a correlation between variables or the effectiveness of a new treatment. Beginning circa 1925, Sir Ronald Fisher—an English statistician, evolutionary biologist, geneticist, and eugenicist (shown in )—standardized the interpretation of statistical significance, and was the main driving force behind the popularity of tests of significance in empirical research, especially in the social and behavioral sciences.
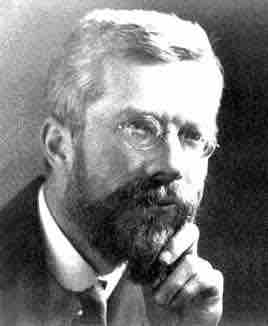
Sir Ronald Fisher
Sir Ronald Fisher was an English statistician, evolutionary biologist, geneticist, and eugenicist who standardized the interpretation of statistical significance (starting around 1925), and was the main driving force behind the popularity of tests of significance in empirical research, especially in the social and behavioral sciences.
Statistical significance refers to two separate notions:
- the
$p$ -value, (the probability that the observed data would occur by chance in a given true null hypothesis); or - the Type I error rate
$\alpha$ (false positive rate) of a statistical hypothesis test (the probability of incorrectly rejecting a given null hypothesis in favor of a second alternative hypothesis).
In relation to Fisher, statistical significance is a statistical assessment of whether observations reflect a pattern rather than just chance. The fundamental challenge is that any partial picture of a given hypothesis, poll or question is subject to random error. In statistical testing, a result is deemed statistically significant if it is so extreme (without external variables which would influence the correlation results of the test) that such a result would be expected to arise simply by chance only in rare circumstances. Hence the result provides enough evidence to reject the hypothesis of "no effect. "
Reading Tests of Significance
A typical test of significance comprises two related elements:
- the calculation of the probability of the data, and
- an assessment of the statistical significance of that probability.
Probability of the Data
The probability of the data is normally reported using two related statistics:
- a test statistic (
$z$ ,$t$ ,$F$ …), and - an associated probability (
$p$ ,$^*$ ).
The information provided by the test statistic is of little immediate usability and can be ignored in most cases. The associated probability, on the other hand, tells how probable the test results are and forms the basis for assessing statistical significance.
Statistical Significance
The statistical significance of the results depends on criteria set up by the researcher beforehand. A result is deemed statistically significant if the probability of the data is small enough, conventionally if it is smaller than 5% (
As an example, consider the following test statistics:
In this example, the test statistics are