When putting together a hypothesis test, consideration of directionality is critical. The vast majority of hypothesis tests involve either a point hypothesis, two-tailed hypothesis or one-tailed hypothesis. A one-tailed test or two-tailed test are alternative ways of computing the statistical significance of a data set in terms of a test statistic, depending on whether only one direction is considered extreme (and unlikely) or both directions are considered extreme. The terminology "tail" is used because the extremes of distributions are often small, as in the normal distribution or "bell curve" . If the test statistic is always positive (or zero), only the one-tailed test is generally applicable, while if the test statistic can assume positive and negative values, both the one-tailed and two-tailed test are of use.
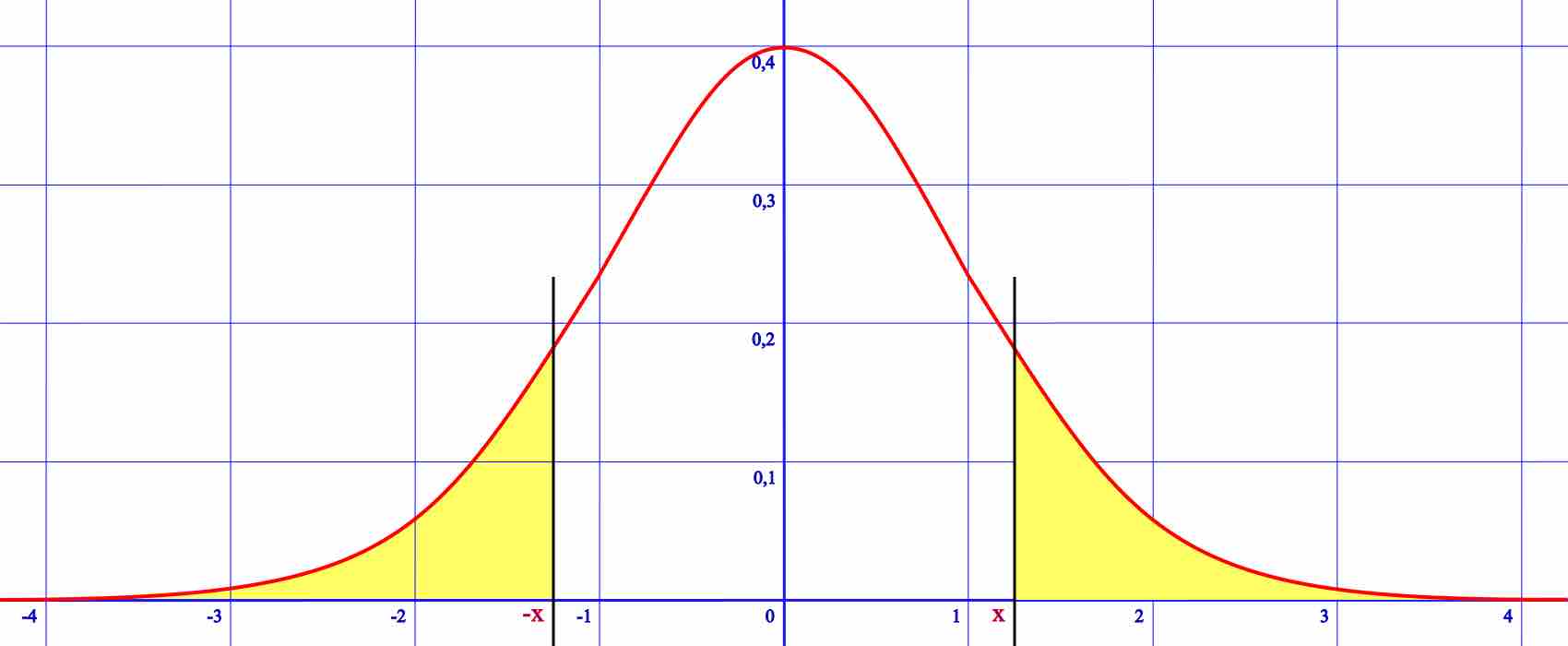
Two-Tailed Test
A two-tailed test corresponds to both extreme negative and extreme positive directions of the test statistic, here the normal distribution.
A one-tailed hypothesis is a hypothesis in which the value of a parameter is specified as being either:
- above or equal to a certain value, or
- below or equal to a certain value.
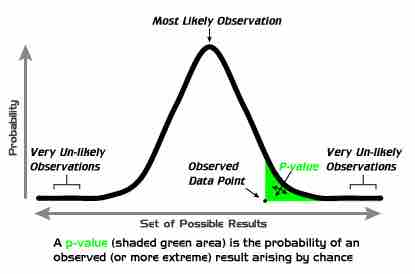
One-Tailed Test
A one-tailed test, showing the
An example of a one-tailed null hypothesis, in the medical context, would be that an existing treatment,
Applications of One-Tailed Tests
One-tailed tests are used for asymmetric distributions that have a single tail (such as the chi-squared distribution, which is common in measuring goodness-of-fit) or for one side of a distribution that has two tails (such as the normal distribution, which is common in estimating location). This corresponds to specifying a direction. Two-tailed tests are only applicable when there are two tails, such as in the normal distribution, and correspond to considering either direction significant.
In the approach of Ronald Fisher, the null hypothesis
For example, if flipping a coin, testing whether it is biased towards heads is a one-tailed test. Getting data of "all heads" would be seen as highly significant, while getting data of "all tails" would not be significant at all (