Facts About Population Proportions
You do not need to be a math major or a professional statistician to have an intuitive appreciation of the following:
- In order to estimate the proportions of some attribute within a population, it would be helpful if you could rely on the proportions observed within a sample of the population.
- If you want to rely on a sample, it is important that the sample be random. This means that the sampling was done in such a way that each member of the underlying population had an equal chance of being selected for the sample.
- The size of the sample is important. As the size of a random sample increases, there is greater "confidence" that the observed sample proportion will be "close" to the actual population proportion. If you were to toss a fair coin ten times, it would not be that surprising to get only 3 or fewer heads (a sample proportion of 30% or less). But if there were 1,000 tosses, most people would agree – based on intuition and general experience – that it would be very unlikely to get only 300 or fewer heads. In other words, with the larger sample size, it is generally apparent that the sample proportion will be closer to the actual "population" proportion of 50%.
- While the sample proportion might be the best estimate of the total population proportion, you would not be very confident that this is exactly the population proportion.
Finding the Population Proportion Using Confidence Intervals
Let's look at the following example. Assume a political pollster samples 400 voters and finds 208 for Candidate
where
Often, statisticians like to use specific confidence intervals for
where
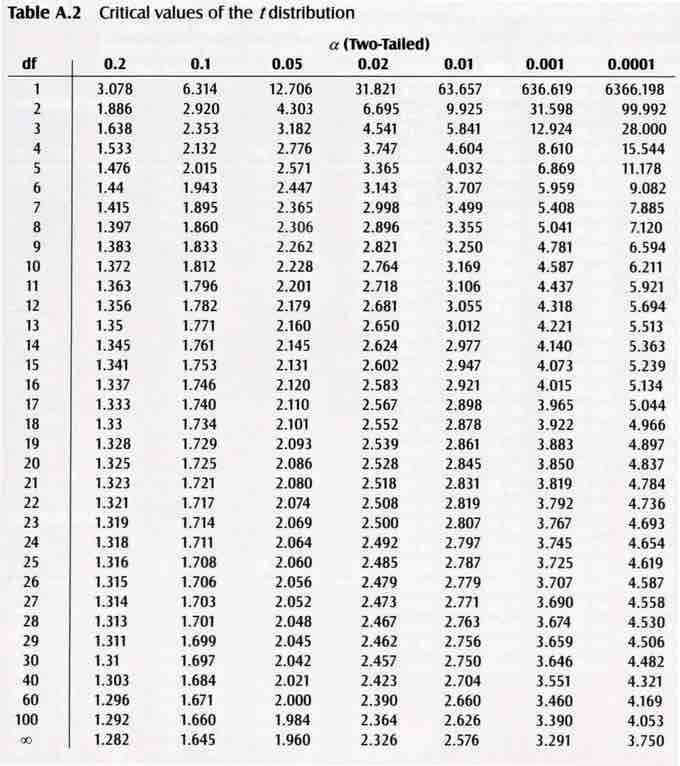
Critical Value Table
A simple guideline – If you use a confidence level of