Cohen's
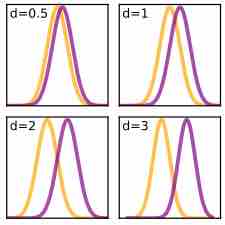
Cohen's $d$
Plots of the densities of Gaussian distributions showing different Cohen's effect sizes.
The concept of effect size already appears in everyday language. For example, a weight loss program may boast that it leads to an average weight loss of 30 pounds. In this case, 30 pounds is an indicator of the claimed effect size. Another example is that a tutoring program may claim that it raises school performance by one letter grade. This grade increase is the claimed effect size of the program. These are both examples of "absolute effect sizes," meaning that they convey the average difference between two groups without any discussion of the variability within the groups.
Reporting effect sizes is considered good practice when presenting empirical research findings in many fields. The reporting of effect sizes facilitates the interpretation of the substantive, as opposed to the statistical, significance of a research result. Effect sizes are particularly prominent in social and medical research.
Cohen's
As in any statistical setting, effect sizes are estimated with error, and may be biased unless the effect size estimator that is used is appropriate for the manner in which the data were sampled and the manner in which the measurements were made. An example of this is publication bias, which occurs when scientists only report results when the estimated effect sizes are large or are statistically significant. As a result, if many researchers are carrying out studies under low statistical power, the reported results are biased to be stronger than true effects, if any.
Relationship to Test Statistics
Sample-based effect sizes are distinguished from test statistics used in hypothesis testing in that they estimate the strength of an apparent relationship, rather than assigning a significance level reflecting whether the relationship could be due to chance. The effect size does not determine the significance level, or vice-versa. Given a sufficiently large sample size, a statistical comparison will always show a significant difference unless the population effect size is exactly zero. For example, a sample Pearson correlation coefficient of
Cohen's D
Cohen's
Cohen's
The precise definition of the standard deviation s was not originally made explicit by Jacob Cohen; he defined it (using the symbol