The calculated statistical significance of a result is in principle only valid if the hypothesis was specified before any data were examined. If, instead, the hypothesis was specified after some of the data were examined, and specifically tuned to match the direction in which the early data appeared to point, the calculation would overestimate statistical significance.
Testing Hypotheses Suggested by the Data
Testing a hypothesis suggested by the data can very easily result in false positives (type I errors) . If one looks long enough and in enough different places, eventually data can be found to support any hypothesis. Unfortunately, these positive data do not by themselves constitute evidence that the hypothesis is correct. The negative test data that were thrown out are just as important, because they give one an idea of how common the positive results are compared to chance. Running an experiment, seeing a pattern in the data, proposing a hypothesis from that pattern, then using the same experimental data as evidence for the new hypothesis is extremely suspect, because data from all other experiments, completed or potential, has essentially been "thrown out" by choosing to look only at the experiments that suggested the new hypothesis in the first place.
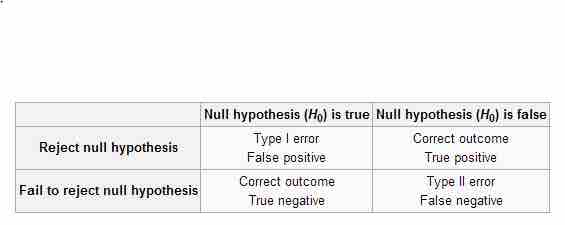
Types of Errors
This table depicts the difference types of errors in significance testing.
A large set of tests as described above greatly inflates the probability of type I error as all but the data most favorable to the hypothesis is discarded. This is a risk, not only in hypothesis testing but in all statistical inference as it is often problematic to accurately describe the process that has been followed in searching and discarding data. In other words, one wants to keep all data (regardless of whether they tend to support or refute the hypothesis) from "good tests", but it is sometimes difficult to figure out what a "good test" is. It is a particular problem in statistical modelling, where many different models are rejected by trial and error before publishing a result.
The error is particularly prevalent in data mining and machine learning. It also commonly occurs in academic publishing where only reports of positive, rather than negative, results tend to be accepted, resulting in the effect known as publication bias..
Data Snooping
Sometimes, people deliberately test hypotheses once they've seen the data. Data snooping (also called data fishing or data dredging) is the inappropriate (sometimes deliberately so) use of data mining to uncover misleading relationships in data. Data-snooping bias is a form of statistical bias that arises from this misuse of statistics. Any relationships found might appear valid within the test set but they would have no statistical significance in the wider population. Although data-snooping bias can occur in any field that uses data mining, it is of particular concern in finance and medical research, which both heavily use data mining.